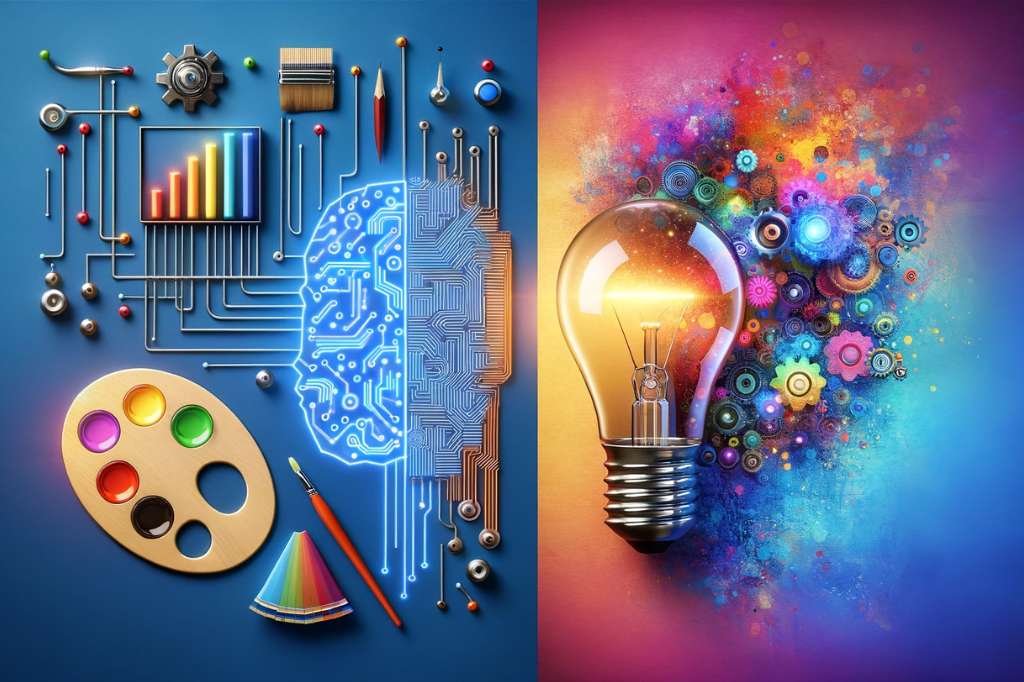
AI vs Generative AI: Key Differences Explained
Artificial Intelligence (AI) has become ubiquitous in our lives, from the personalized recommendations on your shopping app to the AI-powered assistants in our homes. But there’s a new buzzword in town: Generative AI.
Before we delve deeper, let’s quickly understand that AI primarily focuses on analyzing and making decisions based on existing data, while Generative AI takes a step further by creating new, original content inspired by learned data.
While both fall under the umbrella of AI, they have distinct personalities and exciting potentials. So, what exactly is the difference, and how will they shape the future?
Actually, it’s a bit too premature to ask how they would shape the future. However, as regulations on use of AI are enacted in all countries, the impact would be clearer.
What is AI (Artificial Intelligence)?
Think of AI as the ultimate data detective. It excels at analyzing data to identify patterns, trends, and anomalies. This analytical prowess translates into numerous applications:
- Predicting the future: AI algorithms can analyze past data to predict future trends, helping businesses optimize inventory, understand customer behaviour, and even predict potential equipment failures.
- Making informed decisions: AI can analyze massive amounts of data in real-time, enabling businesses and individuals to make data-driven decisions with greater accuracy and efficiency.
- Automating repetitive tasks: From chatbots answering customer queries to self-driving cars navigating traffic, AI automates tasks, freeing up human time and resources for more complex endeavours.
However, AI has limitations. It typically thrives on structured data and struggles with the nuances of human language and the complexities of creative thinking. This is where generative AI steps in.
What is Generative AI?
Imagine an AI that can not only analyze data but also create new things based on its knowledge. That’s the magic of generative AI. It takes inspiration from existing data and uses various techniques to generate entirely new content, including:
- Realistic images: From generating portraits that don’t exist to creating photorealistic landscapes, generative AI can push the boundaries of image creation.
- Compelling text: News articles, poems, even scripts and musical pieces – generative AI can create human-readable and even engaging content in various formats.
- Sound and music: From composing original melodies to mimicking specific artists’ styles, generative AI is making waves in the audio domain.
Generative AI vs AI
So, how does generative AI achieve these feats? Here are some key players:
- Generative Adversarial Networks (GANs): Imagine two AI systems playing a game. One, the generator, tries to create new data (like an image) that appears real. The other, the discriminator, attempts to distinguish the generated data from real data. This constant “adversarial” battle pushes both systems to improve, leading to increasingly realistic and sophisticated outputs.
- Variational Auto Encoders (VAEs): These models act like data compression and decompression tools. They first compress the data into a latent space, capturing its essence. Then, they attempt to reconstruct the data from this compressed representation, allowing for the generation of similar but novel data points.
Generative AI Applications
Generative AI’s potential extends far beyond creating cool images or writing poems. Here are a few exciting real-world applications:
- Drug discovery: By analyzing existing data on molecules and their properties, generative AI can suggest potential new drug candidates, accelerating the process of drug discovery.
- Materials science: Similar to drug discovery, generative AI can suggest novel materials with desired properties, leading to advancements in fields like battery technology and sustainable materials development.
- Personalized learning: Generative AI can create personalized learning materials and adapt to individual student needs, paving the way for a more engaging and effective learning experience.
Challenges and Considerations
While AI and generative AI offer immense potential, they also come with challenges:
- Bias and fairness: If trained on biased data, these models can perpetuate and amplify existing societal biases. Careful data selection and ongoing monitoring are crucial to mitigate this risk.
- Misinformation and deep-fakes: The ability to create realistic synthetic media raises concerns about the spread of misinformation and deep-fakes. Robust ethical frameworks and technical advancements are needed to address this.
- Overreliance and creativity: Overdependence on generative AI for content creation can stifle human creativity and critical thinking. Finding the right balance between AI and human expertise is crucial for responsible development.
The Future of AI and Generative AI
The future of AI and generative AI lies in collaboration. Combining their strengths – AI’s analytical prowess and generative AI’s creative power – can unlock new possibilities:
- Enhanced AI capabilities: Generated data can be used to train more robust AI models and explore new areas of application.
- Human-AI collaboration: As AI and generative AI evolve, fostering an environment where humans and machines work together to leverage each other’s strengths will be paramount.
- Unlocking creativity and innovation: The combined power of AI and generative AI can open doors for groundbreaking discoveries and new forms
- Unlocking creativity and innovation: The combined power of AI and generative AI can open doors for groundbreaking discoveries and new forms of creative expression. Imagine an AI-powered design tool that can generate a multitude of design options based on user input, or a music composition tool that helps musicians experiment with new sounds and styles.
Need for Responsible Development
As we move forward, it’s crucial to ensure responsible development and ethical considerations are at the forefront:
- Transparency and Justification: We need to understand how these models work and what data they are based on to build trust and mitigate potential biases.
- Regulation and governance: Clear guidelines and regulations are necessary to address concerns surrounding deepfakes, misinformation, and potential misuse of these technologies.
- Education and awareness: Public education and awareness campaigns are crucial to foster understanding and responsible use of AI and generative AI.
Conclusion
AI and generative AI are powerful tools with the potential to revolutionize various aspects of our lives. By understanding their unique strengths and limitations, fostering responsible development, and promoting human-AI collaboration, we can harness their potential to create a better future for all.
Remember, the future of these technologies lies in our hands – let’s work together to ensure it’s a future filled with progress, creativity, and responsible innovation. As the world of AI evolves, we can see more advances in Generative AI and its current systems. We will also see a rise in the use of both, AI and Generative AI in the coming days.